Exclusive Web Stories)– Machine Learning Architecture in the Age of Artificial Intelligence
- Due to rapid technological advancement, machine learning (ML) is becoming more crucial in Artificial Intelligence research.
- This article digs into the details of Machine Learning Architecture in the Age of Artificial Intelligence, discussing its importance, its components, and the role it will play in creating the future of artificial intelligence.
Also Read:
Hands-On Artificial Intelligence for Banking, A New Era in Finance
1. Intro
Artificial intelligence (AI) has revolutionized our relationship with digital devices.
Machine learning, a branch of Artificial Intelligence, is pivotal because of its meteoric rise in importance.
In this article, we’ll look at how machine learning was built and why it’s so important in the era of Artificial Intelligence.
2. Understanding Machine Learning Architecture in the Age of Artificial Intelligence
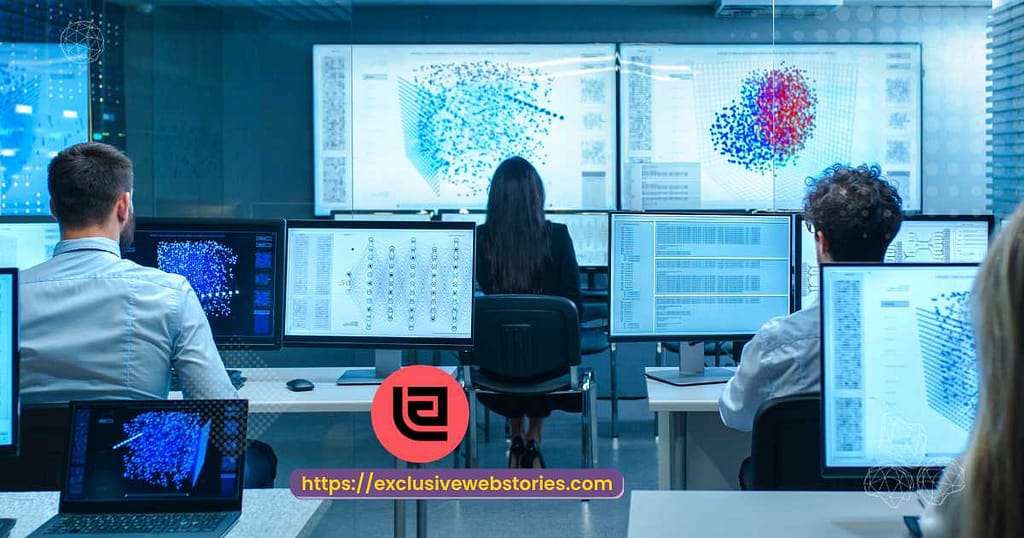
2.1 What is Machine Learning?
In artificial intelligence, machine learning is the study and creation of systems with the ability to learn from data and act accordingly. It allows computers to learn how to get better at a specific task over time without any additional instruction.
2.2 Types of Machine Learning
Supervised learning, unsupervised learning, and reinforcement learning are the three main branches of machine learning. Each one is better suited to a certain task, such as prediction or finding previously unseen patterns in data.
Also Read:
Artificial Intelligence and Cybersecurity Opportunities and Challenges
3. The Evolution of ML Architecture
3.1 Traditional ML vs. Modern ML
Traditional approaches to machine learning have given way to more cutting-edge practices as the field has progressed.
Modern ML uses neural networks and deep understanding to automatically extract features from data, whereas classical ML relies on created features and techniques.
3.2 Neural Networks and Deep Learning
Brain-like neural networks have had a profound effect on the field of machine learning.
Advances in areas like picture and speech recognition have been made possible by deep understanding, a type of neural network that allows computers to interpret large amounts of unstructured data.
4. Components of Machine Learning Architecture
4.1 Data Collection and Preprocessing
Machine learning cannot function without data. In this step, data is gathered, cleaned, and organized in preparation for analysis.
In order to effectively train ML models, high-quality data is required.
4.2 Model Building and Training
Several methods and architectural frameworks are used to construct machine learning models.
In order for a model to learn patterns and make predictions, it must be trained with data.
4.3 Evaluation and Fine-Tuning
Models are tested for accuracy and efficiency after training is complete. To increase the model’s accuracy in prediction, fine-tuning is an iterative process.
5. Applications of ML Architecture in Artificial Intelligence
Machine learning architecture has many potential uses in many fields, including medicine and finance.
It’s the driving force behind everything from diagnostics in healthcare to self-driving cars.
6. Challenges and Considerations
6.1 Data Quality and Quantity
The efficiency of ML models might vary widely depending on the quality and quantity of available data.
Maintaining information that is both accurate and complete is a constant struggle.
6.2 Ethical Concerns
Concerns about prejudice, privacy, and fairness have emerged as ML becomes more widespread. Responsible Artificial Intelligence development requires attention to these concerns.
7. The Future of Machine Learning Architecture
7.1 Advancements in ML
With developments in fields like explainable Artificial Intelligence, quantum computing, and federated learning, the future of ML architecture looks bright.
7.2 Integration with Artificial Intelligence Systems
The development of Artificial Intelligence relies heavily on ML. The technology will become more advanced and self-sufficient once it is combined with AI systems.
8. Benefits of ML Architecture
Advantages of machine learning architecture include automation, enhanced decision-making, and the ability to deal with complicated and extensive data sets.
Also Read:
How to Manage Powertec Workbench Multi System Exercise Chart
Bottom Line:
The foundation of artificial intelligence is machine learning architecture, which drives progress in many different areas.
To succeed in a world increasingly dominated by artificial intelligence, knowledge of its building blocks and uses is crucial.